multitemporal analysis
Change detection methods for remote sensing
Multitemporal analysis of satellite data is of utmost importance for different earth observation applications. Our team develops data-driven methods for multitemporal analysis of very high resolution satellite data.
Selected related publications
- In Papadomanolaki et al. 2021 we present a UNet-like architecture which models the temporal relationship of spatial feature representations using integrated fully convolutional LSTM blocks on top of every encoding level.
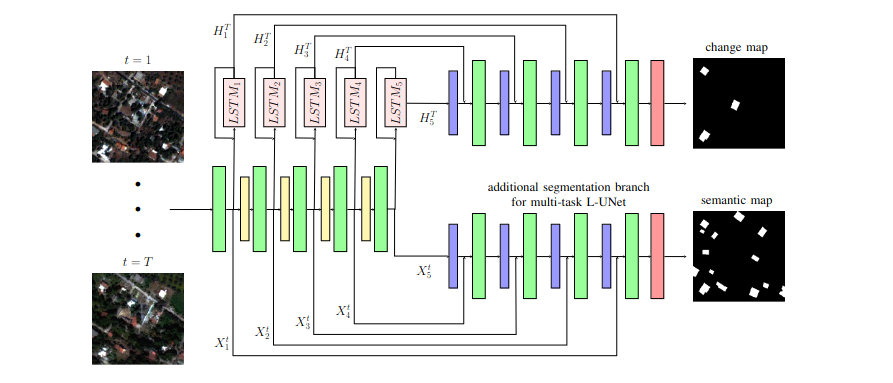
Overview of the method presented in the Trasaction on Geoscience and Remote Sensing 2021 [Papadomanolaki et al. 2021]
- In Papadomanolaki et al. 2019 presents a novel deep learning framework for urban change detection which combines state-of-the-art fully convolutional networks for feature representation and powerful recurrent networks for temporal modelling of Sentinel-2 multitemporal data.
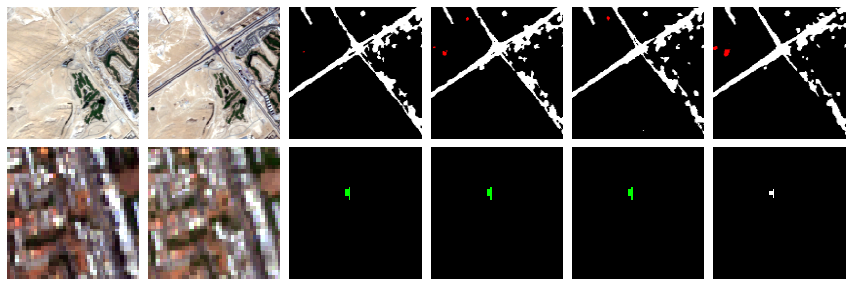
Results presented in the IGARSS 2019 [Papadomanolaki et al. 2019]
References
2021
- A deep multitask learning framework coupling semantic segmentation and fully convolutional LSTM networks for urban change detectionIEEE Transactions on Geoscience and Remote Sensing, 2021
2019
- Detecting urban changes with recurrent neural networks from multitemporal Sentinel-2 dataIn IGARSS 2019-2019 IEEE international geoscience and remote sensing symposium, 2019